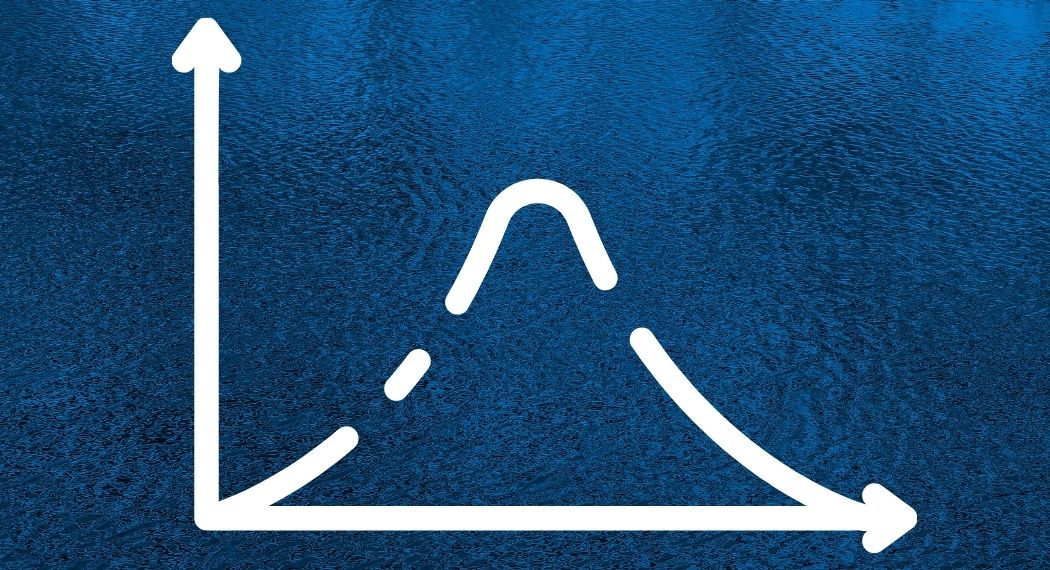
Seminario de Probabilidad y Estadística
Título: Solving Inverse Problems in Imaging by Posterior Sampling with Auto-Encoding Prior
Expositor: Mario Gonzalez (DMEL-Cenur Litoral Norte)
Resumen: In Bayesian statistics, prior knowledge about the unobserved signal of interest is expressed as a prior distribution which, combined with observational data in the form of a likelihood function allows to determine the posterior distribution. This posterior can be used to derive point estimates such as the MAP or MMSE estimators, but also to estimate uncertainty in these predictions, e.g. in the form of confidence intervals. Most of the work using generative models such as Generative Adversarial Networks (GAN) or Variational AutoEncoders (VAE) as image priors focus on computing point estimates. On the other hand, MCMC methods for sampling from the posterior distribution permit the exploration of the solution space and computing point estimates as well as other statistics about the solutions such as uncertainty estimates. However, the performance of widely used methods like Metropolis-Hastings depends on having precise proposal distributions which can be challenging to define in high-dimensional spaces. In this talk, we present a Gibbs-like posterior sampling algorithm that exploits the bidirectional nature of VAE networks. Thanks to the GPU's parallelization capability, we efficiently run multiple chains which explore more rapidly the posterior distribution and also give more accurate convergence tests. To accelerate the burn-in period we explore the adaptation of the annealed importance sampling with resampling method.
Viernes 28/10 a las 10:30
unicamente por zoom
Contacto: Alejandro Cholaquidis - acholaquidis [at] hotmail.com (acholaquidis[at]hotmail[dot]com)
La charla será únicamente de forma virtual.
https://salavirtual-udelar.zoom.us/j/81121640094?pwd=SWVsZ1V2TTI5aDZob0NTdXVRVzhVZz09
Página del seminario: https://pye.cmat.edu.uy/seminario
Página del grupo: https://pye.cmat.edu.uy/home
Canal de youtube: https://www.youtube.com/channel/UCOPZEOrLSAYPz2qCAL-KqMg/about